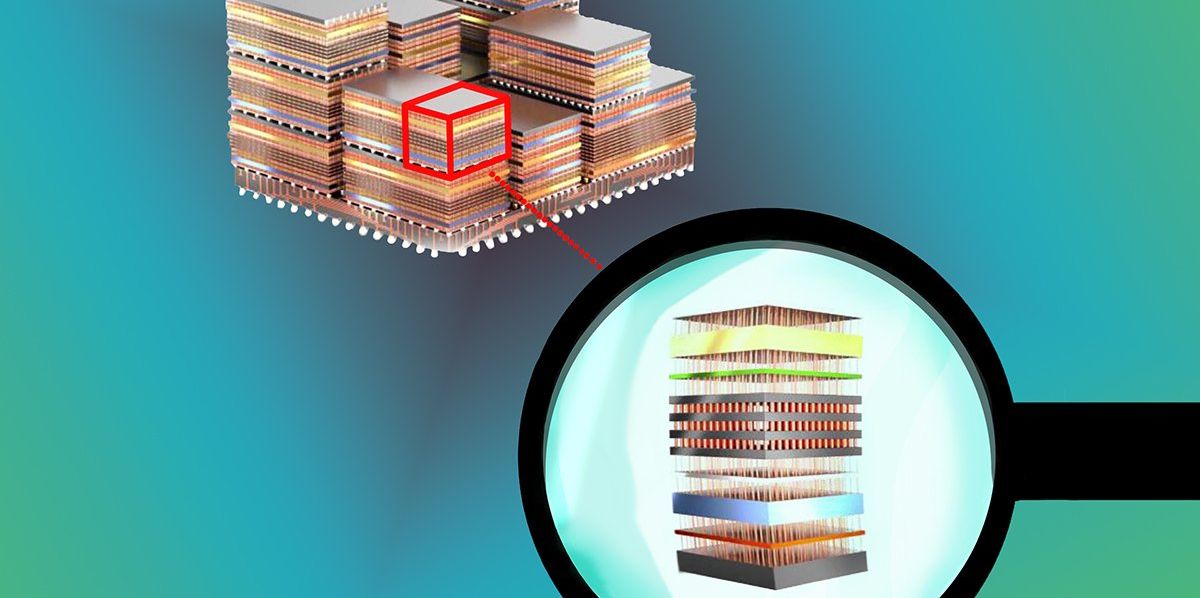
The way forward for reminiscence is huge, various, and tightly built-in with processing. That was the message of an invited talk this week on the International Electron Devices Meeting in San Francisco. H.-S. Philip Wong, {an electrical} engineer at Stanford College and former vice chairman of analysis at TSMC who stays a scientific advisor to the corporate, instructed the assembled engineers it’s time to consider reminiscence and computing structure in new methods.
The calls for of immediately’s computing issues—notably AI—are quickly outpacing the capabilities of present reminiscence programs and architectures. Right now’s software program assumes it’s potential to randomly entry any given little bit of reminiscence. Transferring all that knowledge forwards and backwards from reminiscence to processor consumes time and great quantities of power. And all the info shouldn’t be created equal. A few of it must be accessed continuously, some occasionally. This “reminiscence wall” downside requires a reckoning, Wong argued.
Sadly, Wong mentioned, engineers proceed to give attention to the query of which rising know-how will exchange the traditional reminiscence of immediately, with its hierarchy of SRAM, DRAM, and Flash. Every of those applied sciences—and their potential replacements—has tradeoffs. Some present quicker readout of knowledge; others present quicker writing. Some are extra sturdy; others have decrease energy necessities. Wong argued that searching for one new reminiscence know-how to rule all of them is the unsuitable method.
“You can not discover the right reminiscence,” Wong mentioned.
As an alternative, engineers ought to take a look at the necessities of their system, then choose and select which mixture of parts will work finest. Wong argued for ranging from the calls for of particular kinds of software program use instances. Choosing the right mixture of reminiscence applied sciences is “a multidimensional optimization downside,” he mentioned.
One Reminiscence Can’t Match All Wants
Making an attempt to make one form of reminiscence one of the best at all the pieces—quick reads, quick writes, reliability, retention time, density, power effectivity, and so forth—leaves engineers “working too onerous for no good motive,” he mentioned, exhibiting a slide with a picture of electrical-engineer-as-Sisyphus, pushing a gear up a hill. “We’re taking a look at numbers in isolation with out understanding what a know-how goes for use for. These numbers could not even matter,” he mentioned.
For example, when designing a system that might want to do frequent, predictable reads of the reminiscence and rare writes, MRAM, PCM, and RRAM are good selections. For a system that might be streaming a excessive quantity of knowledge, the system wants frequent writes, few reads, and solely requires a brief knowledge lifetime. So engineers can commerce off retention for pace, density, and power consumption and go for achieve cells or FeRAM.
With discuss of beginning up nuclear power vegetation to gas AI knowledge facilities, it’s clear the business is conscious of its power downside.
This sort of flexibility can result in nice advantages. For instance, Wong factors to his work on hybrid gain cells, that are much like DRAM however use two transistors in every reminiscence cell as an alternative of a transistor and a capacitor. One transistor is silicon and offers quick readout; the opposite shops the info with no need refreshing, and relies on an oxide semiconductor. When these hybrid achieve cells are mixed with RRAM for AI/machine studying coaching and inference, they supply a 9x power use profit in contrast with a conventional reminiscence system.
And crucially, mentioned Wong, these various reminiscences have to be extra intently built-in with computing. He argued for integrating a number of chips, every with their very own native reminiscences, in an “phantasm system” that treats every of those chips as a part of one bigger system. In a paper printed in October, Wong and his collaborators suggest a computing system that makes use of a silicon CMOS chip as its base, layered with fast-access dense reminiscence made up of STT-MRAM, layers of metallic oxide RRAM for non-volatile reminiscence, and layers of excessive pace, excessive density achieve cells. They name this a MOSAIC (MOnolithic, Stacked, and Assembled IC). To avoid wasting power, knowledge will be saved close to the place it will likely be processed, and chips within the stack will be turned off once they’re not wanted.
Throughout the query session, an engineer within the viewers mentioned that he liked the thought however famous that each one these totally different items are made by totally different firms that don’t work collectively. Wong replied that this is the reason conferences like IEDM that convey everybody collectively are vital. With discuss of beginning up nuclear energy vegetation to gas AI knowledge facilities, it’s clear the business is conscious of its power downside.“Necessity is the mom of invention,” he added.
From Your Website Articles
Associated Articles Across the Internet