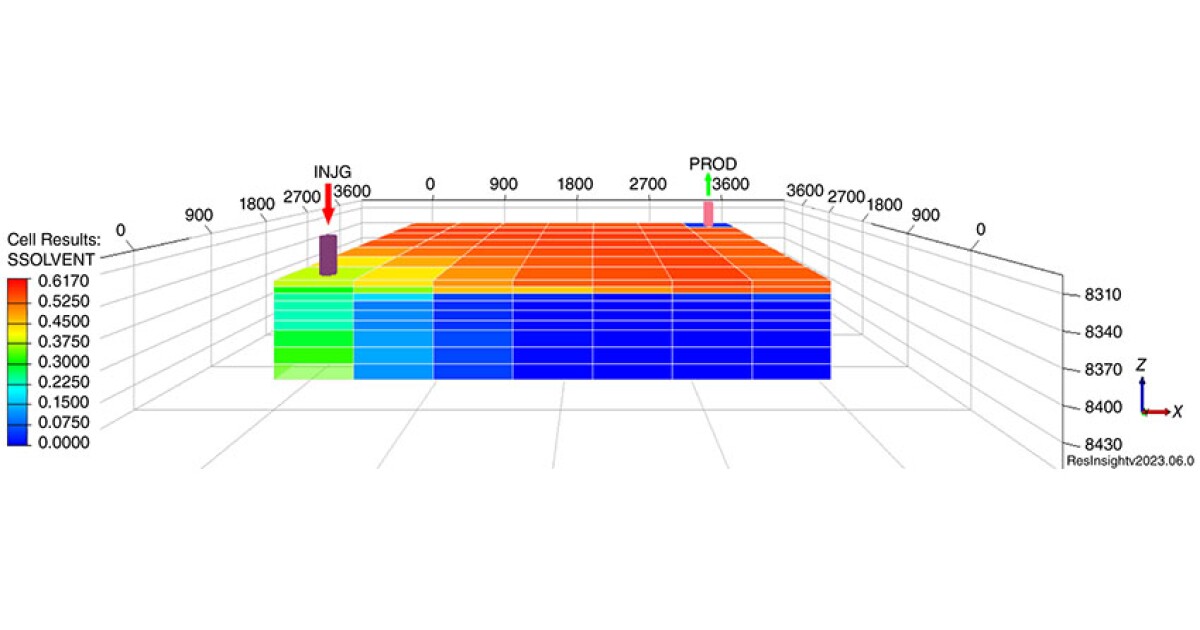
An open-source framework is introduced for the event and analysis of machine learning- (ML) assisted data-driven fashions of CO2 enhanced oil restoration (EOR) processes to foretell oil manufacturing and CO2 retention. The primary goal of the authors was to extend the velocity, robustness, and accuracy of predicting oil restoration and CO2 retention utilizing a whole open-source method combining Python programming, reservoir simulation, and ML strategies.
Overview of the Open-Supply Framework
The analysis of the predictive fashions was carried out utilizing two CO2 water-alternating-gas (WAG) simulation circumstances, which have been proposed utilizing the SPE Comparative Answer Venture (CSP) 5 simulation mannequin as a reference. First, a reservoir simulation deck template and a configuration file, together with variable inputs, have been generated to create any variety of simulation jobs for every case. The input-value vary for the simulations was set in a Python script.