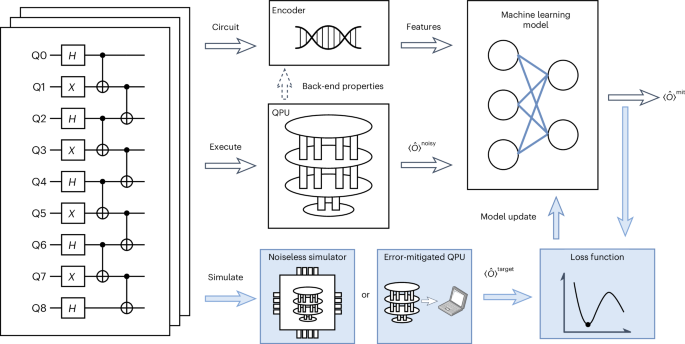
Biamonte, J. et al. Quantum machine learning. Nature 549, 195–202 (2017).
Daley, A. J. et al. Practical quantum advantage in quantum simulation. Nature 607, 667–676 (2022).
Campbell, E. T., Terhal, B. M. & Vuillot, C. Roads towards fault-tolerant universal quantum computation. Nature 549, 172–179 (2017).
Bravyi, S., Dial, O., Gambetta, J. M., Gil, Darío & Nazario, Z. The future of quantum computing with superconducting qubits. J. Appl. Phys. 132, 160902 (2022).
Cai, Z. et al. Quantum error mitigation. Rev. Mod. Phys. 95, 045005 (2023).
Kandala, A. et al. Error mitigation extends the computational reach of a noisy quantum processor. Nature 567, 491–495 (2019).
van den Berg, E., Minev, Z. K., Kandala, A. & Temme, K. Probabilistic error cancellation with sparse Pauli–Lindblad models on noisy quantum processors. Nat. Phys. 19, 1116–1121 (2023).
Kim, Y. et al. Scalable error mitigation for noisy quantum circuits produces competitive expectation values. Nat. Phys. 19, 752–759 (2023).
Kim, Y. et al. Evidence for the utility of quantum computing before fault tolerance. Nature 618, 500–505 (2023).
Temme, K., Bravyi, S. & Gambetta, J. M. Error mitigation for short-depth quantum circuits. Phys. Rev. Lett. 119, 180509 (2017).
Li, Y. & Benjamin, S. C. Efficient variational quantum simulator incorporating active error minimization. Phys. Rev. X 7, 021050 (2017).
Tsubouchi, K., Sagawa, T. & Yoshioka, N. Universal cost bound of quantum error mitigation based on quantum estimation theory. Phys. Rev. Lett. 131, 210601 (2023).
Quek, Y. et al. Exponentially tighter bounds on limitations of quantum error mitigation. Nat. Phys. 20, 1648–1658 (2024).
Takagi, R., Tajima, H. & Gu, M. Universal sampling lower bounds for quantum error mitigation. Phys. Rev. Lett. 131, 210602 (2023).
Kim, C., Park, K. D. & Rhee, J.-K. Quantum error mitigation with artificial neural network. IEEE Access 8, 188853–188860 (2020).
Czarnik, P., Arrasmith, A., Coles, P. J. & Cincio, L. Error mitigation with Clifford quantum-circuit data. Quantum 5, 592 (2021).
Czarnik, P., McKerns, M., Sornborger, A.T. & Cincio, L. Improving the efficiency of learning-based error mitigation. Preprint at https://arxiv.org/abs/2204.07109 (2022).
Bennewitz, E. R., Hopfmueller, F., Kulchytskyy, B., Carrasquilla, J. & Ronagh, P. Neural error mitigation of near-term quantum simulations. Nat. Mach. Intell. 4, 618–624 (2022).
Patel, T. & Tiwari, D. QRAFT: reverse your quantum circuit and know the correct program output. In Proc. 26th ACM International Conference on Architectural Support for Programming Languages and Operating Systems 443–455 (2021).
Strikis, A., Qin, D., Chen, Y., Benjamin, S. C. & Li, Y. Learning-based quantum error mitigation. PRX Quantum 2, 040330 (2021).
Shtanko, O. et al. Uncovering local integrability in quantum many-body dynamics. Preprint at https://arxiv.org/abs/2307.07552v1 (2023).
Huang, H.-Y. et al. Power of data in quantum machine learning. Nat. Commun. 12, 2631 (2021).
Huang, H.-Y., Kueng, R., Torlai, G., Albert, V. V. & Preskill, J. Provably efficient machine learning for quantum many-body problems. Science 377, eabk3333 (2022).
Ezzell, N., Pokharel, B., Tewala, L., Quiroz, G. & Lidar, D. A. Dynamical decoupling for superconducting qubits: a performance survey. Phys. Rev. Applied 20, 064027 (2023).
Pokharel, B. & Lidar, D. A. Demonstration of algorithmic quantum speedup. Phys. Rev. Lett. 130, 210602 (2023).
Seif, A. et al. Suppressing correlated noise in quantum computers via context-aware compiling. In 51st Annual International Symposium on Computer Architecture 310–324 (ISCA, 2024).
Bennett, C. H. et al. Purification of noisy entanglement and faithful teleportation via noisy channels. Phys. Rev. Lett. 76, 722 (1996).
Wallman, J. J. & Emerson, J. Noise tailoring for scalable quantum computation via randomized compiling. Phys. Rev. A 94, 052325 (2016).
Hashim, A. et al. Randomized compiling for scalable quantum computing on a noisy superconducting quantum processor. Phys. Rev. X 11, 041039 (2021).
van den Berg, E., Minev, Z. K. & Temme, K. Model-free readout-error mitigation for quantum expectation values. Phys. Rev. A 105, 032620 (2022).
Lowe, A. et al. Unified approach to data-driven quantum error mitigation. Phys. Rev. Res. 3, 033098 (2021).
Kingma, D. P. & Ba, J. Adam: a method for stochastic optimization. Preprint at https://arxiv.org/abs/1412.6980 (2015).
Ying, R. et al. Graph convolutional neural networks for web-scale recommender systems. In Proc. 24th ACM SIGKDD International Conference on Knowledge Discovery & Data Mining 974–983 (2018).
Reiser, P. et al. Graph neural networks for materials science and chemistry. Commun. Mater. 3, 93 (2022).
Shi, Y. et al. Masked label prediction: unified message passing model for semi-supervised classification. In Proc. 13th International Joint Conference on Artificial Intelligence, IJCAI-21 1548–1554 (2021).
Ranjan, E., Sanyal, S. & Talukdar, P. ASAP: adaptive structure aware pooling for learning hierarchical graph representations. In AAAI Conference on Artificial Intelligence (2019).
Rivero, P., Metz, F., Hasan, A., Brańczyk, A. M. & Johnson, C. Zero noise extrapolation prototype. GitHub https://github.com/qiskit-community/prototype-zne (2022).
Sitdikov, I., Minev, Z. K. & Liao, H. Machine learning for practical quantum error mitigation. Zenodo https://doi.org/10.5281/zenodo.13769804 (2024).
Kandala, A. et al. Hardware-efficient variational quantum eigensolver for small molecules and quantum magnets. Nature 549, 242–246 (2017).