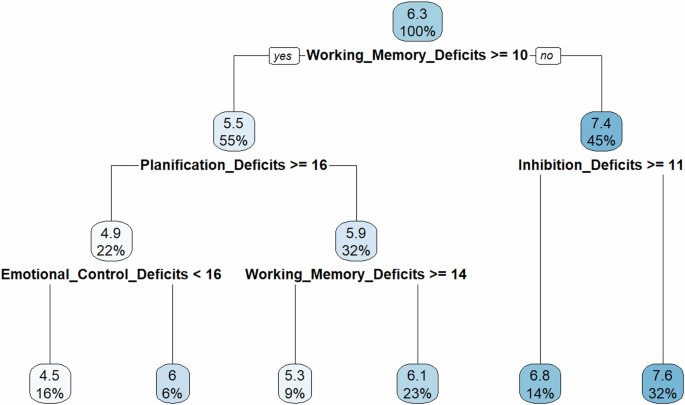
The aim of this study was to use machine learning to identify and characterise profiles of Spanish students in compulsory secondary education, based on their learning outcomes in Language and Literature, on the one hand, and Mathematics, on the other hand, and their levels of executive functions, both cognitive (working memory, inhibition, cognitive flexibility, and planning) and emotional (emotion regulation). The generated regression trees provided these student profiles, offering information on the different interactions and relevance of executive function deficits to their learning results in Language and Literature and Mathematics. Individual differences in both hot and cool executive function skills deficits are related to their learning results in both areas. These results support those from past studies (Zelazo & Carlson 2020).
Regarding Hypothesis 1 (students with a profile characterised by greater difficulties in executive functions (both cognitive and emotional) will achieve poorer learning results in both Spanish Language and Literature and Mathematics, as compared to their peers with profiles characterised by lower executive difficulties, who will achieve superior learning results), the results obtained partially supported this hypothesis. These results indicate that, in both Language and Literature and Mathematics, students achieving the best learning results are those having fewer executive function problems, thus corroborating the hypothesis. Thus, in Language and Literature, participants who achieve the best results (terminal node 6, with an average of 7.6 points) are characterised by not having working memory deficits ≥ 10 and having no inhibition deficits ≥ 11. In Mathematics, participants achieving the best results (terminal node 8, with an average of 7.8 points) are characterised by not having working memory deficits ≥ 10, having no planning deficits ≥ 12, and no inhibition deficits ≥ 9. Therefore, having a lower level of working memory deficits and inhibition deficits is relevant to achieving good performance in both Language and Literature and Mathematics. Furthermore, in Mathematics, having a lower level of planning deficits is also relevant. At the other extreme, in the case of students with the worst learning results in each subject, some results also corroborate the formulated hypothesis, while others do not. Specifically, in Language and Literature, students with the worst learning results (terminal node 1, the only ones who fail, with an average of 4.5 points) display a profile that is characterised by working memory deficits ≥ 10, planning deficits ≥ 16 and emotional control deficits 2013). Considering the other student profiles (nodes 3–6), it is seen that emotional control deficits are not relevant to their learning results. In these profiles where only cold executive function deficits come into play, Hypothesis 1 is corroborated: students whose profile is characterised by greater difficulties in cold executive functions, as compared to their peers, achieve lower learning results. In the case of learning outcomes in Mathematics, for one of the student groups that achieves the worst results (failing the subject (terminal node 2 = average score 4.8)), the situation that appeared in the tree referring to Language and Literature is repeated: with the same profile of difficulties in cold executive functions (terminal nodes 2 and 3), having a lower level of emotional control deficits (terminal node 2 versus terminal node 3) is associated with poorer learning results (terminal node 2 = 4.8 versus terminal node 3 = 6). Thus, once again, with a certain profile of working memory deficits and planning deficits, having or not having a certain level of emotional control deficits (in this case,
Numerous studies have corroborated the fact that in profiles where only cold executive function deficits are implicated, greater difficulties in these cognitive executive functions are associated with lower learning outcomes, and lower difficulties in these executive functions are associated with higher learning outcomes. This relationship holds for students at distinct educational levels, with and without learning difficulties, belonging to various economic backgrounds and nationalities (Zelazo & Carlson 2020). Furthermore, in our results, participants whose profile is determined solely by cold executive functions (thus confirming Hypothesis 1) are the majority of the sample: 78% of participants in the case of Language and Literature = students forming terminal nodes 3–6; 73% in the case of Mathematics = students forming terminal nodes 1, and from 4 to 8. The finding that only cold executive function deficits (but not emotional control deficits) are associated with learning outcomes for most of the sample, is in line with results from past studies in which no relationship was found between emotional control and learning outcomes (Brock et al. 2009). However, and unlike these works, in our study, in some students (in the case of Language and Literature = 22% of participants = students forming terminal nodes 1 and 2; in the case of Mathematics = 27% of participants = students forming terminal nodes 2 and 3; all of them remarkably among those who attained the worst results), emotional control deficits are relevant to determining their learning outcomes. This is consistent with the findings of other works (Ahmed et al. 2013; Álvarez-Huerta et al. 2023; Huang 2023; Kahl et al. 2021; Oberle et al. 2014; Nadeem et al. 2023; Gustems-Carnicer et al. 2019).
Remarkably, however, the importance of the emotional control variable on learning outcomes in our research points in a different direction than in previous research. Numerous studies have indicated that higher levels of emotional control are associated with better learning outcomes, so difficulties in emotional control negatively affect learning results (Graziano et al. 2007; Kwon et al. 2017). However, in our study, the opposite occurs: with the same profile of cognitive executive functions, students with greater difficulties in emotional control obtain better results than those who have a lower level of difficulties in emotional control (which, as previously mentioned, does not corroborate Hypothesis 1). These conflicting results may be due to the precise content of the questionnaire items evaluating emotional control deficits, that is, the type of emotion regulation strategies to which they refer and, therefore, the type of emotion regulation strategies that have been evaluated in this study. Gross and John’s (2003) process model of emotion regulation, the most frequently cited theoretical framework referring to emotion regulation, distinguishes between two broad types of emotion regulation strategies: cognitive reappraisal and expressive suppression. Cognitive reappraisal is an antecedent-focused strategy where an individual consciously alters their thoughts about a situation (modifies the interpretation or appraisal of the event) to alter the emotions attached to it. Expressive suppression is a response-focused strategy and implies hiding and repressing emotions elicited by a specific situation. Expressive suppression is a less effective strategy than cognitive reappraisal since it does not alter the emotional impact of the experience on a cognitive level but rather, represses emotions, causing a cognitive load that can harm learning and academic performance. However, cognitive reappraisal permits a reduction of negative effects of situations and life experiences and is associated with minimal cognitive costs, resulting in better memory and academic performance (Akhtar et al. 2020; Karagiannopoulou et al. 2023; Nadeem et al. 2023; Gustems-Carnicer et al. 2019). Upon analysing the questionnaire items used in this study, it is observed that they refer to the use of the expressive suppression strategy (e.g., “Has outbursts of anger”). Thus, low scores on emotional control deficits (continuing with the example, participants who never have outbursts of anger score 1 on the response scale) could be reflecting frequent use of expressive suppression strategies (could be they never have outbursts of anger because they repress it). As it has mentioned previously, frequent use of expressive suppression strategies implies frequent use of unbeneficial emotion regulation strategies (because the emotional impact of the experience at a cognitive level is not changed but rather repressed, causing a cognitive load), and hence, they obtain lower learning outcomes than their peers who express their emotions, even if they are negative. This is precisely the problem with the use of expressive suppression strategies: they repress and do not manifest both negative and positive emotions, generating a high internal load (since emotions are not eliminated but are left unexpressed, and suppression requires effort), negatively affecting learning outcomes. In short, displaying fewer difficulties in using strategies that do not benefit learning but harm it, may justify the lower level of learning outcomes of these students (Kahl et al. 2021). Having greater difficulties in using non-beneficial emotion regulation strategies may justify higher learning outcomes. Further research is needed, however, to corroborate and explain these results, which, in line with other works, highlight the complex nature of the association between emotional control and academic outcomes (Nadeem et al. 2023).
As for Hypothesis 2 (working memory will be the most influential predictor of learning results across different learning areas—Language and Literature and Mathematics), the obtained results provided comprehensive support for it. Working memory deficits emerged as the most decisive predictor of both Language and Literature and Mathematics learning outcomes. In the generated regression trees, this variable was strategically positioned, dividing the root node at the upper level, emphasising its significance and superior predictive power for academic outcomes in each academic area. These outcomes align with past research indicating that working memory is a substantial predictor of academic performance (Anjariyah et al. 2022; Cirino 2023; Dubuc et al. 2020). This body of evidence suggests that students with deficiencies in this memory system often face challenges, specifically in reading and mathematics. These associations were observed not only across normative development but also in special populations, including students with learning disabilities and gifted students (Anjariyah et al. 2022; Flórez-Durango et al. 2022). In essence, both our findings and the existing literature underscore the relationship between working memory and essential processes that are crucial for learning achievement. This connection is likely due to the fact that working memory serves as a general cognitive resource that is capable of storing and processing various information types, encompassing words, images and abstract concepts. Working memory actively stores information and makes it available for more intricate cognitive activities such as reasoning, learning and resolving school tasks, whether linguistic or mathematical in nature (Bergman & Söderqvist 2017). Ultimately, working memory serves as a crucial cognitive resource in the context of learning (Berkowitz et al. 2022; Ji & Guo 2023).
Regarding the quality and accuracy of the models obtained, the results are similar in both models. Both Language and Literature learning outcomes model and Mathematics learning outcomes model are good. All the metric values obtained are satisfactory, including R2 values. Although initially R2 values could be considered low, the following considerations should be taken into account for an adequate interpretation of these values in particular and the quality and accuracy of both models in general: (1) the metric values obtained must be considered together (Naser & Alavi 2020, 2023). (2) One of the reasons why R2 value may be low is the existence of non-linear relationships (Ozili 2023), which is frequent characteristic in the complex educational field research (Gomes et al. 2021). (3) In relation to the previous, R2 is not an optimal choice to assess the goodness of fit for non-linear models (Ozili 2023). In these cases, different error metrics can be used (Naser & Alavi 2020, 2023). (4) Additionally, in certain sciences such as the social sciences (to which this study belongs), it is not usual to find high values of R2 (Ozili 2023). The social sciences deal with human behaviour or human relationship that is subject to change from time to time. Human behaviour may change due to a lot of factors. Because of this complex and dynamic nature of human behaviour, it is difficult to accurately predict it and, therefore, the R-squared goodness of fit of the models in the social sciences is weakened. For this reason, in social science, a model with R2 values ranging from 0.1 to 0.5 is considered good, provided that most or all of the explanatory variables are statistically significant (Ozili 2023). Consequently, and taking these considerations into account, it can be concluded that the two models obtained in our study are good enough. Other authors, as Ferrara et al. (2015), in their research using also regression trees to analyse academic issues related to the Literacy and Mathematics learning, obtained R2 values very similar to ours.
Our results contribute to a deeper understanding of the intricate relationships existing between executive functions (EF) and learning outcomes. It is important to note that most existing research on the connection between EF and academic performance has focused on kindergarten or primary school students (Dubuc et al. 2020; Kahl et al. 2021), with very few studies incorporating hot EF. To the best of our knowledge, only two studies have investigated the relationship between emotion regulation and adolescent learning outcomes, both conducted within the context of mathematical achievement. Both studies provided evidence supporting this association (Gumora & Arsenio 2002; Oberle et al. 2014; Kahl et al. 2021). Therefore, the consideration of both cold and hot EF in adolescent students and their interaction with learning outcomes is a noteworthy aspect of our study. Addressing adolescents and their learning outcomes is of great significance. It is crucial to recognise that adolescence is a critical period in the life cycle, during which numerous social, personal and emotional changes must be navigated. How these changes are dealt with can impact adolescent learning, making it imperative to pay special attention to these students, their learning processes and their outcomes. Furthermore, in Spain, a significant portion of the adolescent years coincides with the final stage of compulsory education (as with our study participants). Depending on their experiences and levels of success, adolescents may decide whether to continue their studies, significantly impacting not only their personal and social development but also the economic and social progress of the country (OECD 2023). Therefore, ensuring and supporting high-quality secondary education for all adolescents represents a sound investment. The findings of this study contribute to efforts being undertaken in this direction.
It is also noteworthy that this study considered not only the more commonly examined EF in school-based works—working memory and inhibition (Dubuc et al. 2020)—or only the elemental EF—working memory, inhibition and flexibility—but it also considered other more complex cognitive EF such as planning and the hot EF emotional control. The consideration of the cold or cognitive EF, including planning, working memory, inhibition and flexibility, ensures that our study addresses all of the relevant cold EF arising during adolescence (Laureys et al. 2021, 2022). Additionally, incorporating the hot EF emotional control into our research responds to a need highlighted in recent literature on the inclusion of hot executive functions analysis in educational and learning research (Fombouchet et al. 2023, 2024; Pinochet-Quiroz et al. 2022). This more comprehensive approach to EF allows our results to provide a better understanding of the relationship between cold and hot EF and learning outcomes. However, consistent with previous research, our results underscore that these relationships are highly complex and require further investigation (Poon 2018; Zelazo & Carlson 2020).
In educational decision-making, it is crucial to seek methodologies that are precise and assist in resolving issues related to classifying or predicting students’ learning outcomes. This is essential since significant decisions are derived from these processes to optimise their development and learning. In this context, our study stands out methodologically by employing artificial intelligence, specifically machine learning (specifically the regression trees algorithm), to analyse a timely question such as the determinants of individual differences in learning outcomes. The use of decision trees, especially CART, represents an approach in the study of the relationships between executive functions (EF) and learning outcomes that surpasses the limitations of the more commonly used techniques, offering advantages over other analytical models (see Seftor et al. 2021). Notably, these advantages include an ease of understanding of the graphical representation. Therefore, non-expert users such as teachers can effectively use the output to gain insights into the variables influencing the learning outcomes of their students and take measures to enhance them.
Although recently, major growth is taking place with regard to intelligent machine learning systems, their use remains limited and underexplored in the educational field, despite their advantages and potentialities (Liu & Lee 2022; Luan & Tsai 2021; Matzavela & Alepis 2021). These systems permit a better understanding of student performance. Improved knowledge of the factors influencing learning outcomes helps predict student performance, providing more precise guidance and designing academic curricula that are tailored to specific student needs (Darling-Hammond et al. 2020). Over recent years, studying personal determinants (especially psychological ones) of student learning outcomes has been a challenge for educators, policymakers and researchers alike. The rapid expansion of artificial intelligence has transformed this challenge into an achievable goal, as shown in this research.
Despite these significant contributions, the results of this study should be interpreted with caution given its limitations: (1) sample size; (2) non-random sample selection; and (3) use of a single educational institution, limiting the generalisability of the results. Therefore, future studies should expand the sample by randomly selecting participants from a larger number of institutions to obtain a more heterogeneous sample. (4) The study is cross-sectional, which precludes the detection of changes over time in the relationship between executive functions and learning outcomes. This is relevant considering that both executive functions and learning outcomes undergo changes during the adolescent years, and factors associated with learning results in each academic area may differ depending on age. (5) The analyses conducted do not permit the making of causal inferences. These last two limitations demand a longitudinal approach to further investigation. (6) The study does not include all variables affecting learning outcomes in language and mathematics or all executive functions advocated by different theoretical models. Future studies could include other executive functions and personal variables, in addition to family-related and educational context variables that may influence learning outcomes. (7) The use of third-party-informed rating scales to collect data on student executive functions may impact the results. Informant perception may differ from the actual level of executive functions the students possess. Future studies could explore alternative methodologies to assess the executive functions of students, such as performance-based tasks and observational methods. (8) Although they are reliable predictors of school performance, the use of grades as an indicator of learning outcomes has been criticised. The difficulty of tests and assessment tasks used by each teacher and their grading criteria may vary. Future research may use standardised assessment batteries. However, this would result in a lower ecological validity of the study since, in Spain, where this study was conducted, teacher-assigned grades are the official mode of evaluating students’ learning outcomes.
In addition to considering the aforementioned suggestions to overcome some of the study limitations, the following future perspectives have been proposed: (1) consideration of gender perspective: modelling different regression trees based on the student’s gender (i.e., estimating separate regression trees for boys and girls) would be interesting. Although boys and girls may perform equally well in the same academic area, they appear to use distinct sets of cognitive abilities (Blanch & Aluja 2013). Therefore, the relationship between executive functions (EF) and academic performance may differ between genders, especially during adolescence (Dubuc et al. 2020), possibly due to the different brain maturation patterns, influenced by the sex hormones that play a relevant role in this developmental stage. (2) Attention to the participants’ academic year: modelling different regression trees according to the students’ academic year may provide relevant information. As students advance through the academic years, academic difficulty and demands increase, possibly varying the relevance and level of each executive function required to achieve good learning outcomes. There is also evidence suggesting that academic performance significantly decreases during secondary education (Abin et al. 2020; Dubuc et al. 2020; Spanish Ministry of Education and Vocational Training 2023). (3) Consideration of learning outcomes in other subjects. (4) Study of executive functions and learning outcomes from a more molecular perspective: It is essential to consider that, for example, many working memory models assume that this is a multicomponent system (Baddeley et al. 2020), permitting differentiation between verbal and spatial working memory. The same applies to inhibition, an executive function in which various authors distinguish several subprocesses, such as behavioural inhibition, cognitive inhibition or resistance to interfering stimuli (Dempster & Corkill 1999). A similar situation occurs in the academic domain, where both Language and Literature and Mathematics outcomes are determined by different competencies. Therefore, in future studies, it may be interesting to analyse how each of these subprocesses making up each executive function interacts to achieve distinct curricular competencies. (5) Application of other algorithms in the development of decision trees.